The world of the Internet of Things (IoT) naturally produces a massive amount of real-time data, especially in the form of time series telemetry, which is just a fancy way of saying data that’s collected and recorded over time from connected devices like sensors, machines, and vehicles. This constant flow of data opens up exciting opportunities for Generative AI (GenAI) to play an important role across many areas within a typical IoT environment.
In this ongoing series about GenAI in IoT, we’ll take a closer look at the different ways these two powerful technologies overlap and work together. We’ll explore how GenAI can enhance IoT systems, making them smarter, more efficient, and even easier to use.
It’s also worth noting that the market for GenAI in IoT is growing at an impressive pace. Recent studies show that the market size is expected to jump from $1.58 billion in 2024 to $2.03 billion in 2025, reflecting a strong CAGR of 28.1%. This rapid growth clearly shows the rising importance and confidence businesses have in combining these technologies.
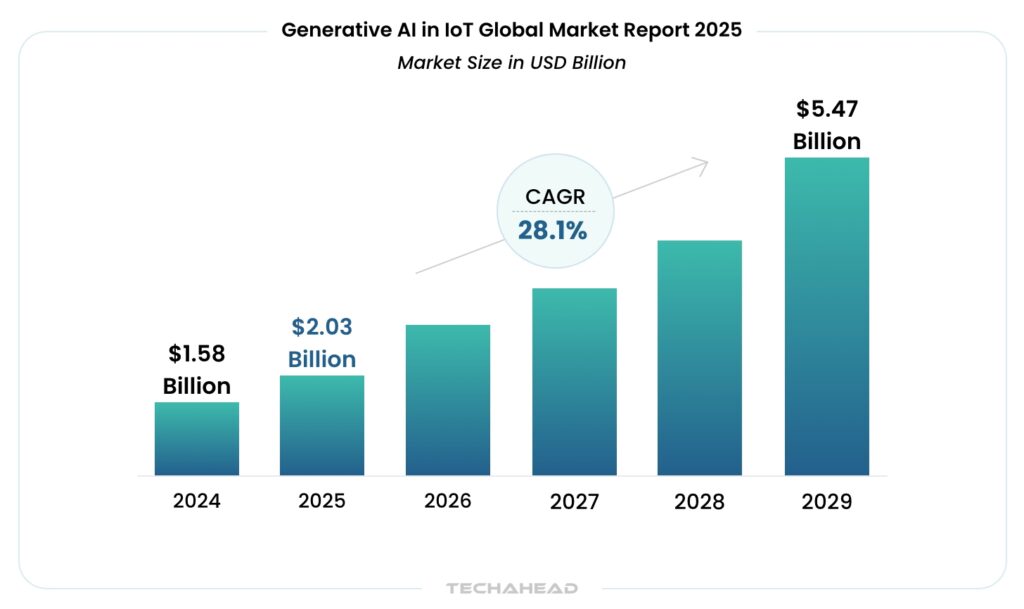
To start things off, this first article will lay the foundation by explaining the basic capabilities and current limitations of Generative AI when applied within the IoT world. Understanding both the potential and the challenges is critical — it helps us make smarter decisions about where, when, and how to use GenAI to truly get the most value out of IoT solutions.
A Winning Combination of GenAI with IoT
When we bring together the Internet of Things (IoT) and Generative AI (GenAI), the result is a powerful synergy that not only addresses their limitations but also unlocks new levels of innovation. Together, these technologies complement each other in ways that make digital ecosystems smarter, more responsive, and highly intuitive.
In simple terms, IoT devices and digital twin technologies can feed real-time, contextual data into GenAI models. This fresh stream of live information helps GenAI operate more accurately, drastically reducing the risk of hallucinations or generating incorrect outputs. In return, GenAI can simplify and interpret the often complex, multi-dimensional data streams from IoT systems, making them easily digestible for end-users through natural language interfaces.
Thus, by working hand-in-hand, IoT and GenAI form a comprehensive, mutually beneficial solution that enhances decision-making, user engagement, and operational efficiency.
Let’s dive deeper into the specific benefits this combination brings:
Natural Language Interaction with Smart Devices
Today, whether at home, in the field, or across manufacturing plants, humans primarily interact with smart devices via digital screens such as smartphones, tablets, and embedded displays. By integrating GenAI’s natural language processing capabilities, these interactions can become far more immersive, intuitive, and human-like. Users can communicate with devices through voice commands or even through emerging technologies like augmented reality (AR) and virtual reality (VR) environments.
For instance, home automation platforms such as Amazon Alexa and Google Home have already pioneered voice-activated assistants. However, with the integration of advanced GenAI models, these interactions will evolve to be even more natural, contextually aware, and emotionally intelligent, significantly elevating the user experience.
Importantly, this seamless natural language interfacing is not confined to consumer gadgets alone. In industries such as manufacturing, engineers can interact directly with machinery through voice-driven digital twins on the shop floor, speeding up maintenance and operational tasks. Similarly, in retail environments, GenAI-powered systems can guide shoppers through stores, answer product inquiries, and assist with billing in real time.
In healthcare, smart conversational agents can offer patients personalized support, answering medical queries, helping them schedule appointments, and even assisting with medication management. The scope for natural language-driven device interaction is vast and still expanding.
Use Cases of Generative AI in IoT
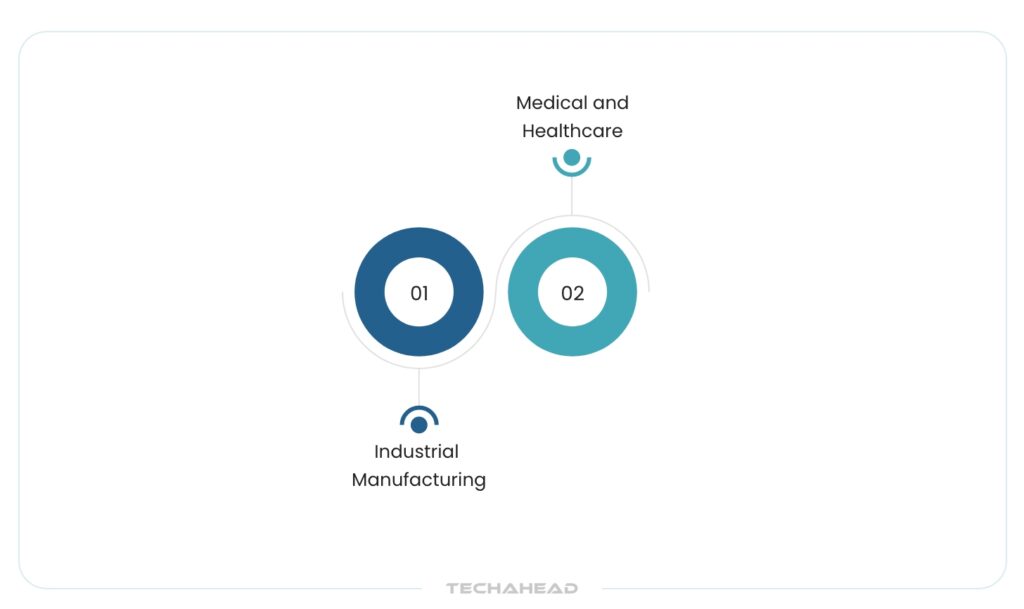
Having explored the potential and limitations of Generative AI (GenAI) in the Internet of Things (IoT), it’s equally important to dive into some of its real-world applications. While this is by no means an exhaustive list, it offers a glimpse into how profoundly GenAI can reshape IoT ecosystems across different industries.
Industrial Manufacturing
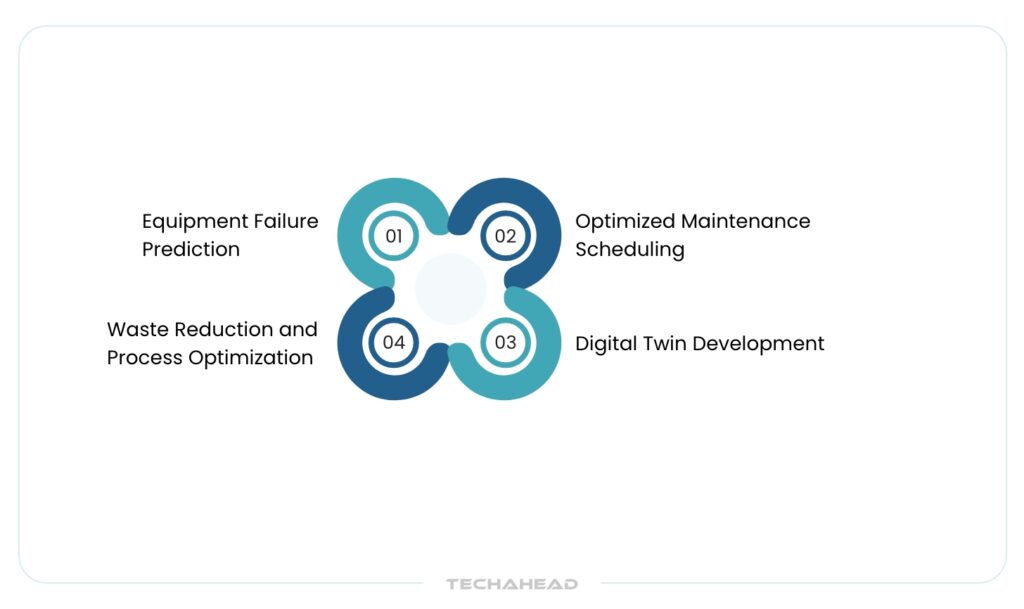
In the realm of industrial manufacturing, GenAI is emerging as a critical enabler of smarter, more efficient operations. When integrated with Large Language Models (LLMs) such as GitHub Copilot, ChatGPT, or Google Bard, GenAI can seamlessly blend real-time telemetry data from equipment with external knowledge sources from the internet. This fusion empowers the system to provide highly contextual recommendations for action, backed by relevant documentation, technical references, and even success probability metrics for different remediation strategies.
Moreover, GenAI serves as a powerful bridge to close the longstanding “data gap” encountered in traditional predictive analytics. In simple terms, where conventional AI models sometimes fall short due to incomplete data or sparse historical records, GenAI can extrapolate and enrich insights by synthesizing available information intelligently.
Some of the key use cases include
- Equipment failure prediction: GenAI can proactively identify early warning signs of equipment or machinery failures, enabling preventive measures before disruptions impact production lines or operational uptime.
- Optimized maintenance scheduling: By accurately forecasting the Remaining Useful Life (RUL) of critical components, GenAI helps companies plan maintenance activities with surgical precision, reducing downtime and maximizing asset utilization.
- Digital twin development: Creating digital replicas of complex systems often requires vast, intricate datasets. GenAI accelerates this process by simulating missing parameters and enhancing model fidelity, which supports better operations planning, performance optimization, and even energy conservation initiatives.
- Waste reduction and process optimization: Through dynamic what-if simulations and advanced analytics, GenAI explores multiple operational scenarios, offering recommendations to minimize waste, reduce inefficiencies, and optimize resource utilization.
Medical and Healthcare
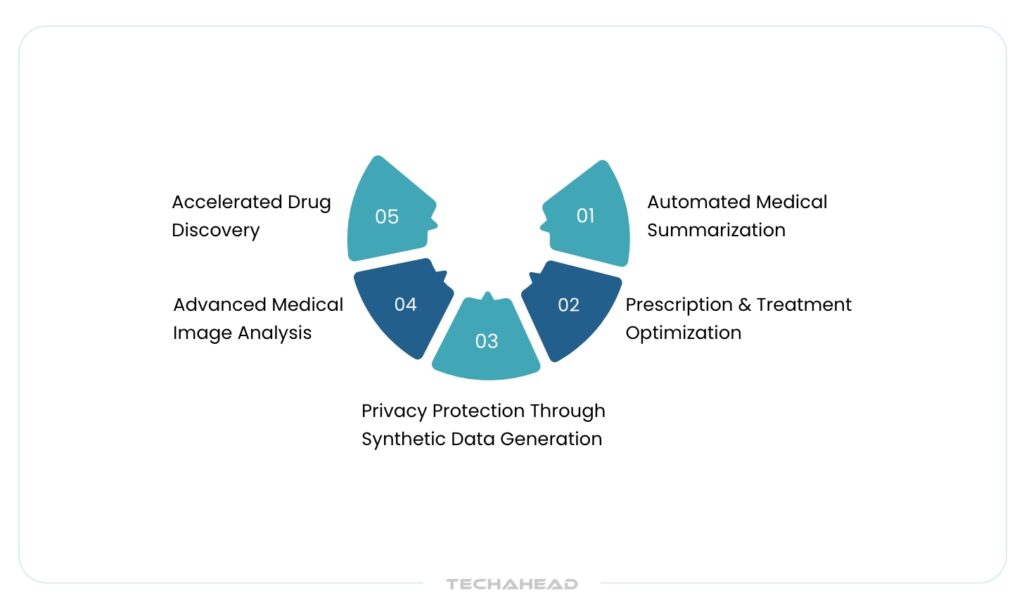
In the healthcare sector, where privacy, accuracy, and efficiency are paramount, GenAI is proving to be a game-changer. From patient data protection to drug discovery, its applications are both broad and impactful.
Key use cases include
- Privacy protection through synthetic data generation: GenAI can create highly realistic, yet fully anonymized, patient datasets that mimic the statistical properties of real-world data. This allows healthcare researchers and AI developers to innovate freely while remaining fully compliant with strict privacy regulations like HIPAA and GDPR.
- Automated medical summarization: Managing and interpreting the flood of data generated by wearables, diagnostic tests, and continuous monitoring devices can be overwhelming. GenAI can distill this data into concise, readable medical summary reports, significantly reducing the administrative burden on healthcare professionals and improving clinical decision-making.
- Prescription and treatment optimization: Based on real-time data from diagnostic tests and wearable devices, GenAI can assist healthcare providers in recommending the most suitable medications and procedures, tailored to the specific needs of each patient.
- Advanced medical image analysis: GenAI enhances traditional imaging techniques by performing tasks like denoising, image reconstruction, and registration on complex medical images, including CT scans, MRIs, ultrasounds, and X-rays. This capability not only improves diagnostic accuracy but also shortens the time required for image interpretation.
- Accelerated drug discovery: The traditional drug development process is notoriously lengthy and expensive. By simulating molecular interactions and predicting biological outcomes, GenAI can significantly speed up the identification and design of new pharmaceutical compounds, potentially bringing life-saving treatments to market faster than ever before.
Limitations of Generative AI in IoT
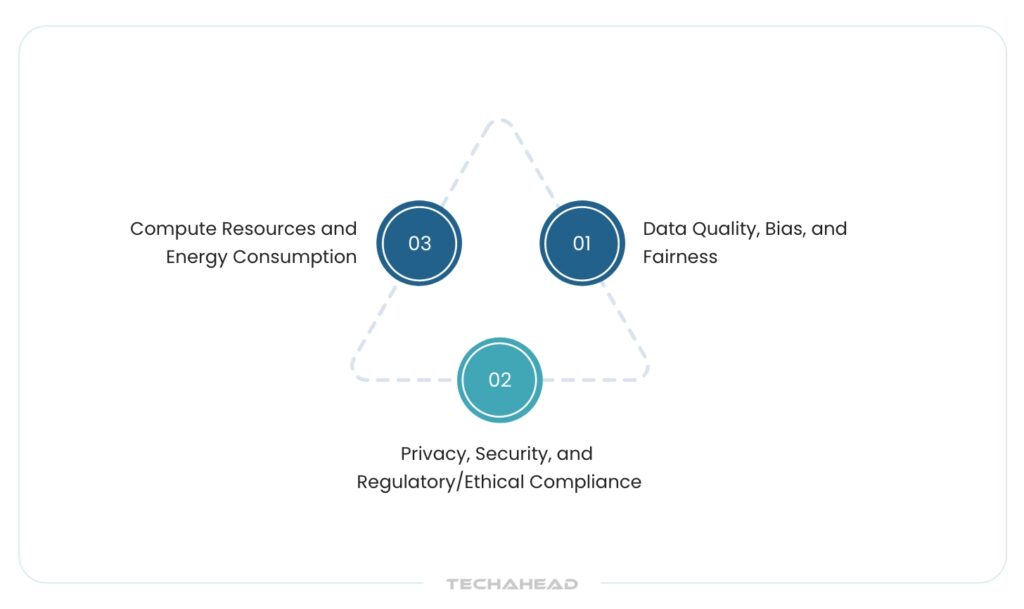
While the integration of generative AI in Internet of Things ecosystem holds tremendous potential, it is essential to recognize and understand its current limitations. Doing so enables companies to make smarter, more informed decisions about how and when to deploy these technologies. Although many of these challenges will gradually diminish as GenAI models evolve, they remain significant considerations today.
Privacy, Security, and Regulatory/Ethical Compliance
Protecting sensitive information remains a major challenge when applying GenAI in IoT environments. Even when data is anonymized or obfuscated, there is still a risk that private information could be inferred or reconstructed, especially given the sophisticated analytics capabilities of modern AI models. Therefore, businesses must conduct thorough vetting and implement rigorous data governance frameworks to mitigate such risks.
Moreover, navigating the complex landscape of regulatory and ethical compliance adds an extra layer of difficulty. Different regions and industries enforce diverse and often stringent regulations around data usage, privacy, and AI ethics. These nuances significantly constrain the deployment of GenAI, particularly in highly regulated sectors like healthcare, finance, and critical infrastructure. As a result, businesses must maintain a proactive and meticulous compliance strategy when integrating GenAI into their IoT operations.
Data Quality, Bias, and Fairness
GenAI models are fundamentally dependent on the quality and diversity of the data they are trained on. In simple terms, if the training data is flawed, biased, or incomplete, the resulting outputs will mirror these imperfections—a phenomenon often summarized as the “garbage in, garbage out” problem.
Given this dependency, deploying GenAI in IoT demands access to vast volumes of clean, representative, and unbiased data. Without such data, there is a high risk that the model will propagate existing biases or generate inaccurate results, leading to skewed decision-making processes. Additionally, ensuring fairness in AI-generated insights becomes particularly critical in applications such as smart cities, healthcare devices, and industrial automation, where outcomes can significantly impact human lives and societal structures. Therefore, organizations must prioritize building robust data pipelines and continuously auditing their datasets for bias and fairness.
Compute Resources and Energy Consumption
Another significant limitation is the intensive computational and energy demands associated with GenAI models. Training these models requires massive processing power, often relying on specialized hardware such as GPUs or TPUs, which translates into high costs and substantial energy consumption.
Even after deployment, the size and complexity of GenAI models pose challenges. They typically require more memory and processing capacity compared to traditional machine learning algorithms. This becomes a major hurdle for IoT devices, many of which operate under strict resource constraints, including limited processing power, minimal storage, and battery-dependent energy supplies.
Consequently, deploying GenAI directly on edge devices, such as sensors, wearables, and industrial IoT equipment, is often impractical without significant model optimization. Techniques like model pruning, quantization, and edge-cloud hybrid approaches are being explored to address these limitations, but they are still evolving. Until these solutions mature, deploying full-scale GenAI in IoT environments will remain a complex balancing act between performance, efficiency, and feasibility.
Conclusion
Now you have built a strong foundation by understanding how Generative AI in IoT fits. It’s time to move a step further. Here, you have understood that the gaps are important because missing or poor-quality data can significantly impact the accuracy and reliability of AI insights.
By identifying the causes early, we can better plan solutions that ensure the success of GenAI applications, whether it’s in smart homes, connected vehicles, industrial automation, or healthcare systems.
As businesses continue to embrace Generative AI in IoT to build smarter, more connected experiences, having the right technical partners becomes essential. Companies looking to integrate these advanced technologies seamlessly into their platforms often collaborate with an experienced mobile app development company, one that not only understands app creation but also the complex needs of IoT and AI ecosystems.
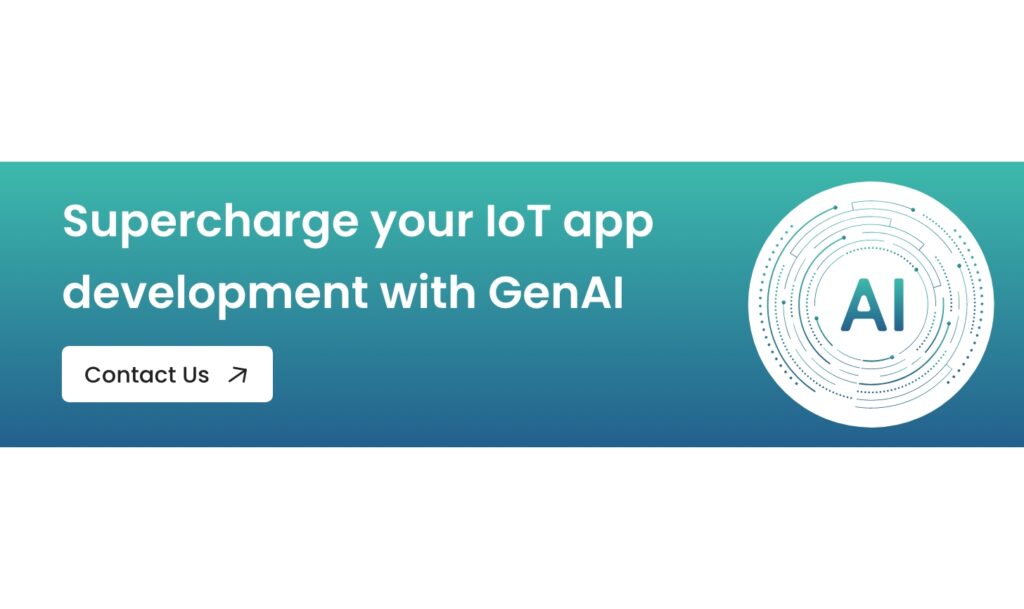
FAQs
Generative AI unlocks new possibilities in IoT by enabling systems to visualize, simulate, and predict outcomes based on real-time sensor data. This powerful combination helps create highly reliable virtual environments, such as those found in the metaverse. By integrating Generative AI with IoT, we can design personalized learning experiences, optimize traffic flow for better decision-making, and even facilitate real-time interactions between users in immersive digital spaces.
Artificial intelligence plays a key role in enhancing the performance and security of IoT devices. AI-driven machine learning algorithms can monitor network traffic, detect unusual activity, and quickly respond to potential cyber threats. This ability to identify and address risks helps strengthen the overall security of IoT ecosystems, making connected devices safer and more resilient.
In the Industrial Internet of Things (IIoT), artificial intelligence brings the power of deep data analysis to the forefront. AI can sift through the vast volumes of data generated by industrial machines and sensors, uncovering patterns and insights that would be impossible for humans to detect alone. This leads to dramatic improvements in operational efficiency, predictive maintenance, and smarter decision-making on the factory floor.
Combining AI with sensors offers significant advantages. AI can process and interpret sensor data with far greater accuracy than traditional methods, leading to more precise readings and better outcomes.
Additionally, AI can optimize the performance of sensors, making them work faster, smarter, and more efficiently. This results in improved system reliability and better overall performance in IoT applications.